You have /5 articles left.
Sign up for a free account or log in.
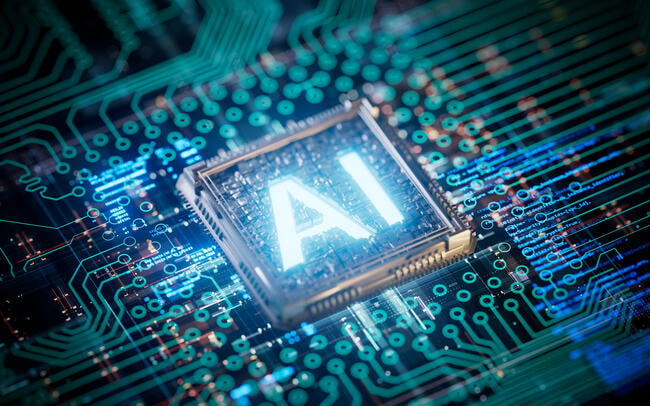
Just_Super/E+/Getty Images
As artificial intelligence rapidly evolves, how can we effectively preserve it?
As a librarian, technologist and community builder who has worked at places like the Internet Archive, the Library of Congress and university research libraries, I see AI preservation as a core challenge that remains to a large extent unaddressed. How can we best understand society moving forward without ongoing access to some version of the tools that fundamentally affect how knowledge in our time is produced?
AI Preservation
Core digital preservation practices like fixity checking, storing geographically distributed copies of data and requiring where possible that data is stored in open formats provide a good foundation for AI preservation efforts.
However, effective AI preservation depends on resolving a number of questions. When we speak of AI preservation, are we referring primarily to preservation in the archival sense (e.g., focus on preserving records of how decisions get made), or are we referring to preservation of models and training data as well?
How should we document AI in support of long-term use? Is Hugging Face’s Model Card approach to describing AI and machine learning (e.g., capturing such characteristics as model type, language, license, bias, risks and limitations) sufficient, or does long-term preservation require a different standard of care?
If training data is essential to evaluation of AI performance, does all training data need to be preserved? Given an uncertain international copyright environment and privacy concerns, should the focus be on preserving information about training data rather than the training data itself? What volume of data should we anticipate preserving? Is bit-level preservation sufficient (this ensures that files do not change, but does not ensure ability to use files within future technical environments), or is emulation required (which entails the use of software to mimic obsolete technical environments)?
What strategies should be employed to offset the environmental impact of AI preservation? How much does AI preservation cost? What sustainability models should be considered to support long-term AI preservation?
What are the significant properties of AI that must be preserved in order for it to be useful down the line?
AI Preservation Partnerships
Given long-standing organizational commitments to AI, librarians at Carnegie Mellon University and the Massachusetts Institute of Technology are actively engaged in projects that can inform AI preservation work. Potential AI preservation efforts can also look to contemporary library-led preservation services and communities like EaaSI (Emulation as a Service Infrastructure), LOCKSS (Lots of Copies Keep Stuff Safe) and the Digital Preservation Coalition. Each speaks to the ability of research libraries to develop policies, practices, communities and infrastructure that ensure long-term preservation and usability of data.
Preservation partnerships between AI service providers and memory organizations like research libraries could help preserve AI. It is a bit of an oversimplification, but AI service providers are generally focused on developing and maintaining cutting-edge, maximally useful versions of tools, while research libraries are generally focused on maintaining multiple versions of things in perpetuity for their intrinsic and/or artifactual value.
This is to say that research libraries provide value to users in part by ensuring long-term access to essential versions of things so that we might better assess the impacts of those things on society during their periods of use. If AI is a core pillar of a Fourth Industrial Revolution, it would seem that we should try to create the most comprehensive and enduring record of that technology and its impacts on society as possible.
Practically, it makes sense to begin with the preservation of open-source AI, though closed-source AI should certainly be part of the work where possible. Perhaps deprecation of closed-source AI could trigger a preservation action. Debates about what constitutes open-source AI continue despite robust efforts to formalize a definition. Reviewing terms of use from self-described open-source AI service providers like Meta and Hugging Face reveals no long-term AI preservation commitments. This does not bode well for future understanding of the now.
There are precedents for cross-sector preservation partnerships that we should be able to learn from. Prior preservation partnerships have done things like send scientific data to a large digital library and code to a European data repository. Particulars of partnerships will vary, but in order to scale they should share in common appropriate levels of financial resourcing from AI service providers for long-term preservation services. Federal policy and investment should also directly address AI preservation needs.
In addition, there are certainly opportunities for philanthropy to play an important role in AI preservation. The Patrick J. McGovern Foundation, the Omidyar Network, the MacArthur Foundation, the Ford Foundation and efforts like Current AI have demonstrated commitments to ensuring responsible development and deployment of AI. It stands to reason that a prerequisite for meeting that objective is ensuring that AI remains available for scrutiny in perpetuity.
We have work to do aligning research libraries, AI service providers, philanthropy and policymakers so they can collaborate on long-term AI preservation. Alignment will be key to resourcing a stable transition from maximally useful AI to an artifactual state available for future study. AI can and must be preserved.